SODAS Lecture: How Machine Learning can Advance Theory Formation in the Social Sciences
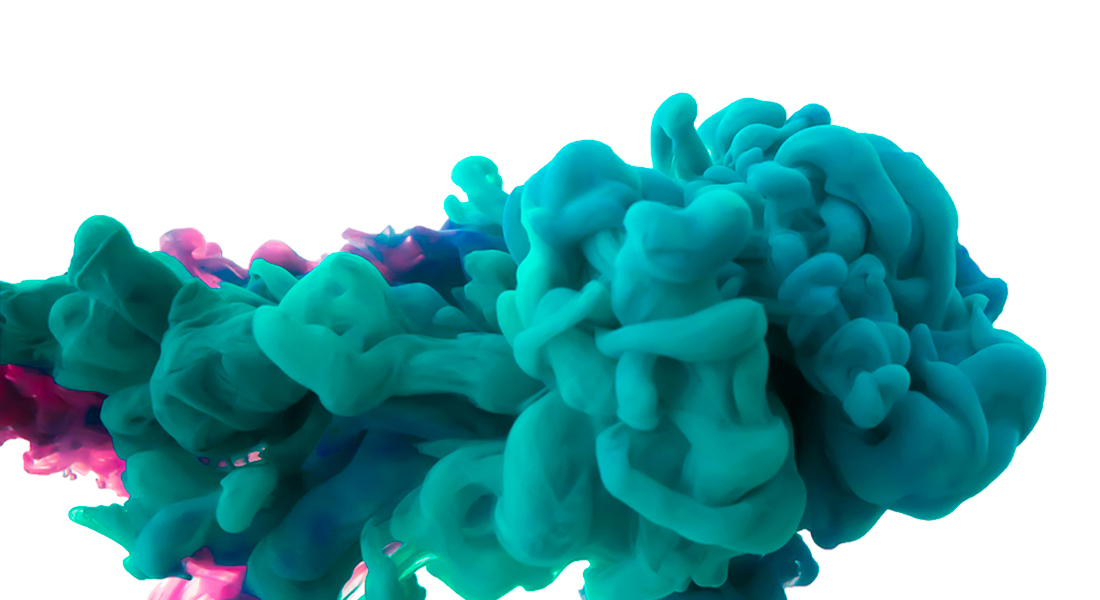
Title:
How Machine Learning can Advance Theory Formation in the Social Sciences
Abstract:
Theories are the vehicle of cumulative knowledge acquisition. At this time, however, many social scientific theories are insufficiently precise to derive testable hypotheses. This limits the advancement of our principled understanding of development. This problem cannot be resolved by improving the way deductive (confirmatory) research is conducted (e.g., through preregistration and replication), because theory formation requires inductive (exploratory) research. In this presentation, I argue that machine learning can help advance theory formation in the social sciences, because it enables rigorous exploration of patterns in data. I will discuss specific advantages of machine learning, explain core methodological concepts, introduce relevant methods, and describe how data-driven insights are consolidated into theory. Machine learning automates exploration, and incorporates checks and balances to ensure generalizable results. It can assist in phenomenon detection and offers a more holistic understanding of the phenomena associated with an outcome or process of interest.
Bio:
Caspar van Lissa is associate professor of social data science at the department of Methodology & Statistics, chair of the Open Science Community Tilburg, and member of the Tilburg Young Academy. His research addresses the epistemological implications of machine learning for theory formation in the social sciences, evidence synthesis – summarizing existing research using machine learning-informed meta-analysis and text mining systematic review – and open reproducible science. He is an advocate for open source research software and has (co-)authored ten R-packages.