SODAS Data Discussion 18 March 2022
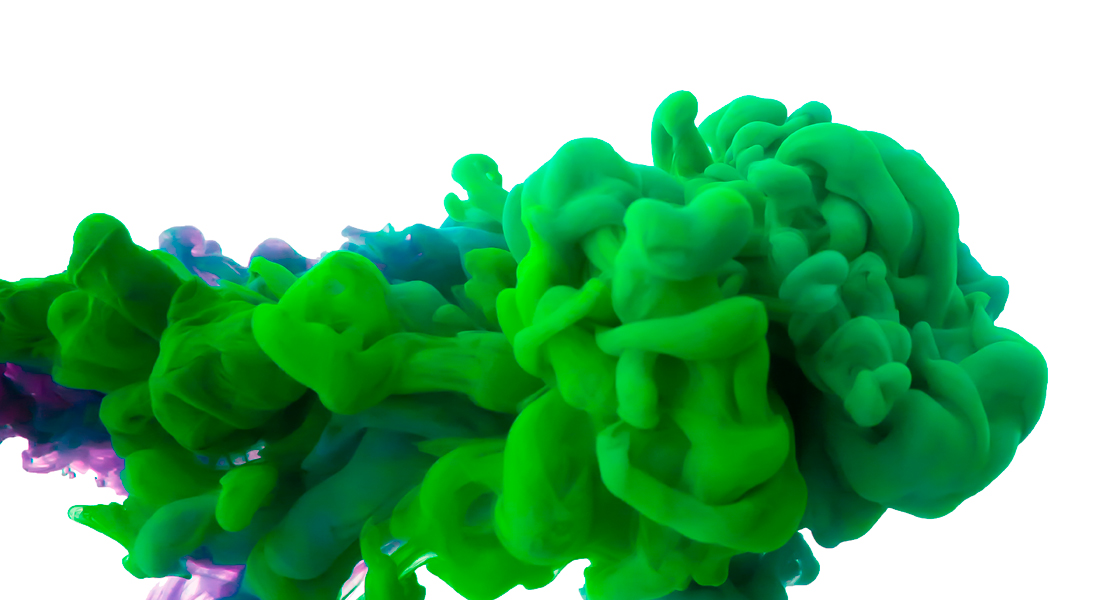
Copenhagen Center for Social Data Science (SODAS) aspirers to be a resource for all students and researchers at the Faculty of Social Sciences. We therefore invite researchers across the faculty to present ongoing research projects, project applications or just a loose idea that relates to the subject of social data science.
The rules are simple: short research presentations of ten minutes are followed by twenty minutes of debate. No papers will be circulated beforehand, and the presentations cannot be longer than five slides.
Author:
Ole Teutloff, PhD, SODAS, University of Copenhagen
Title:
Show me how you look and I tell you what you are worth: Visual measures of human capital
Abstract:
In this research project (idea), we plan to use profile pictures of online freelancers to construct visual measures of human capital. We plan to structure our research as follows. Firstly, we use Convolutional Neural Networks (CNN) to predict hourly wages from profile pictures without any further assumptions. Thereby, we examine to what extent our model can learn how appearance matters for hourly wages without any directive by the researchers of what to focus on. Secondly, we extract various features from the pictures (e.g. gender, ethnicity, age, attractiveness etc.), combine them with non-visual background features (such as education and work experience) and use them to predict the hourly wages of freelancers. To extract the visual features, we train our CNN on publicly available image datasets and evaluate the performance using a professional service such as Face++. Lastly, we combine extracted features, background variables and the profile pictures into one prediction model, and evaluate the gains of using the full image. To further investigate the importance of the extracted features (e.g. gender, ethnicity, attractiveness, education, experience), we also pursue a regression strategy with embeddings created by the CNN. This allows us to evaluate how much the image adds to the prediction on top of the features that we have previously identified and extracted.
- - - - - - - - - - - - - - - - - - - - - - - - - - - - - - - - - - - - - - - - - - - - - - - - - - - - - - - - - - - -
Author:
Simon Polichinel von der Maase, PhD, Department of Political Science, University of Copenhagen
Title:
ConflictNet 1.0 - A probabilistic recurrent Unet for global conflict forecasting.
Abstract:
I here presented a Recurrent (approximate Bayesian) Unet for global conflict forecasting. The network is able to capture the spatial and temporal patterns inherent in violent conflicts and use these patterns to generate forecastings of future conflict patterns. The network works well for both regression and classification task (i.e. estimating the probability of conflict and the magnitude of conflict). It is also able to effectively generate a posterior distribution thus quantifying the model uncertainty.
Venue: CSS, Sodas conference room 1.2.26
or via Zoom: https://ucph-ku.zoom.us/j/64036059160