SODAS Data Discussion 16 September 2022
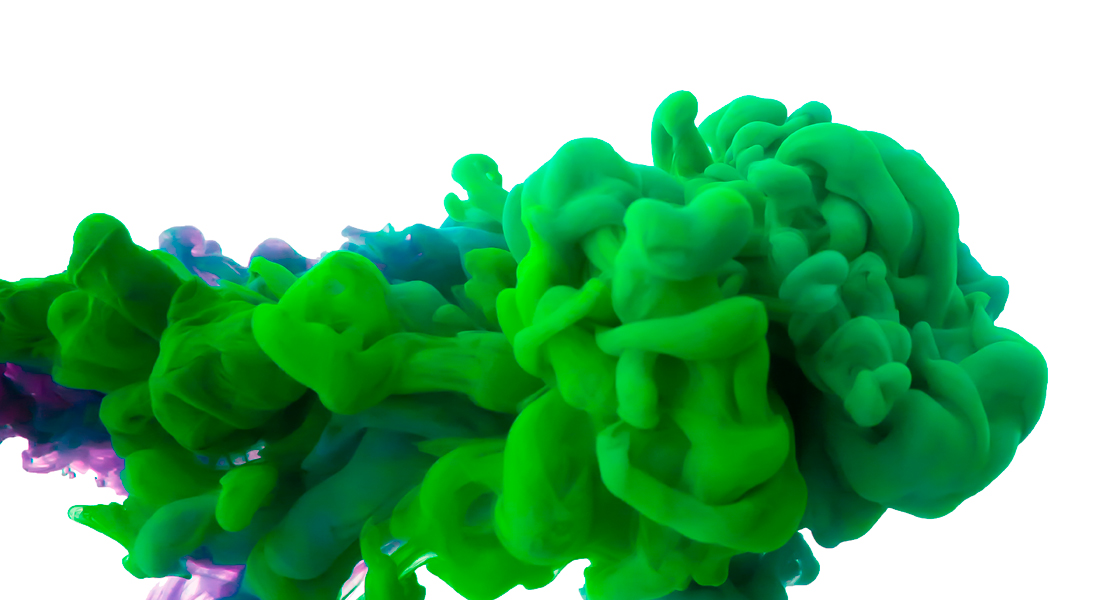
Copenhagen Center for Social Data Science (SODAS) aspirers to be a resource for all students and researchers at the Faculty of Social Sciences. We therefore invite researchers across the faculty to present ongoing research projects, project applications or just a loose idea that relates to the subject of social data science.
The rules are simple: short research presentations of ten minutes are followed by twenty minutes of debate. No papers will be circulated beforehand, and the presentations cannot be longer than five slides.
Presenter: Martin Lund Trinhammer, Department of Psychology, University of Copenhagen (co-authored work with A.C. Holst Merrild, J.F. Lotz and G. Makransky)
Title: Predicting crime during or after psychiatric care: Evaluating machine learning for risk assessment using the Danish patient registries
Abstract: Structural changes in psychiatric systems have altered treatment opportunities for patients in need of mental healthcare. These changes are possibly associated with an increase in post-discharge crime, reported in the increase of forensic psychiatric populations. As current risk-assessment tools are time-consuming to administer and offer limited accuracy, this study aims to develop a predictive model designed to identify psychiatric patients at risk of committing crime leading to a future forensic psychiatric treatment course.
We utilized the longitudinal quality of the Danish patient registries, identifying the 45.720 adult patients who had contact with the psychiatric system in 2014, of which 474 committed crime leading to a forensic psychiatric treatment course after discharge. Four machine learning models (Logistic Regression, Random Forest, XGBoost and LightGBM) were applied over a range of sociodemographic, judicial, and psychiatric variables.
This study achieves a F1-macro score of 76%, with precision = 57% and recall = 47% reported by the LightGBM algorithm. Our model was therefore able to identify 47% of future forensic psychiatric patients, while making correct predictions in 57% of cases. The study demonstrates how a clinically useful initial risk-assessment can be achieved using machine learning on data from patient registries. The proposed approach offers the opportunity to flag potential future forensic psychiatric patients, while in contact with the general psychiatric system, hereby allowing early intervention initiatives to be activated.
- - - - - - - - - - - - - - - - - - - - - - - - - - - - - - - - - - - - - - - - - - - - - - - - - - - - - - - - - - -
Presenters: Andreas Bjerre-Nielsen, Martin Benedikt Busch, Helene Willadsen and Ingo Zettler, Department of Economics, Department of Psychology and SODAS, University of Copenhagen.
Title: Network knowledge and cooperation
Abstract: The ability to recognize cooperators is necessary for sustaining the high levels of cooperation characteristic of human societies. A large body of literature, using laboratory experiments, has explored the role of reputation by unveiling individuals’ previous behavior. These experiments show that individuals with a cooperative reputation are often chosen as partners in settings that allow cooperation. Yet, existing literature has not comprehensively answered how people select cooperative partners when lab-based reputation is unavailable. Using three data inputs, we wish to test the hypothesis that network knowledge increases the ability to choose cooperative partners. First, we use administrative data from the University of Copenhagen alongside Statistics Denmark. Second, we use a survey to elicit the network of at least two TA classes from different study programs. Third, we conduct a Prisoner’s Dilemma experiment with partner choice to investigate cooperativeness and the ability to choose cooperating partners.
In this data discussion, we will primarily present our lab experiment.