SODAS Data Discussion 13 October 2023
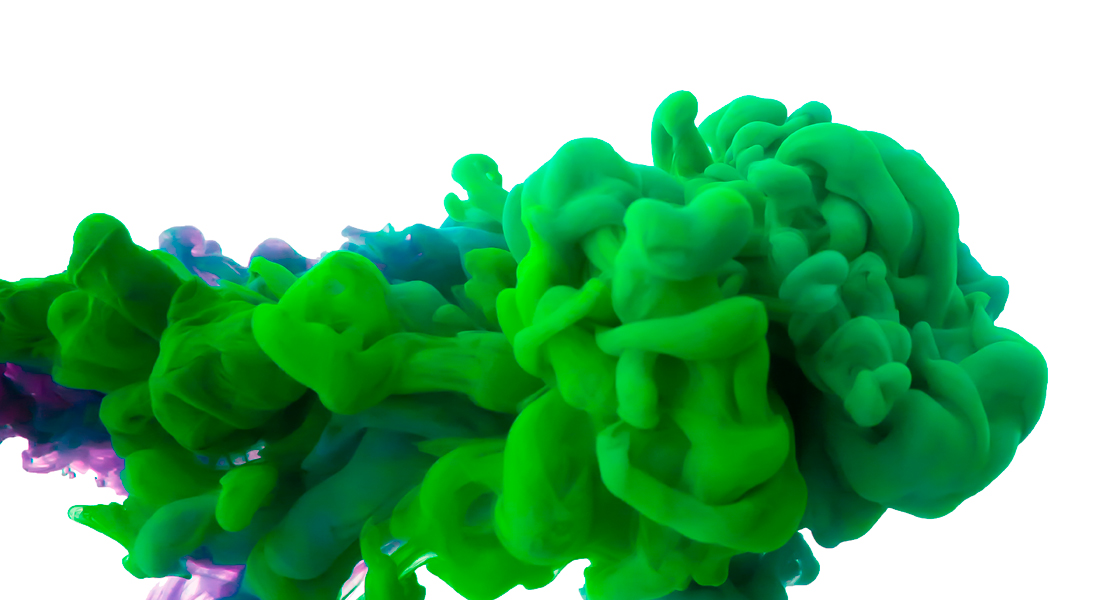
Copenhagen Center for Social Data Science (SODAS) aspirers to be a resource for all students and researchers at the Faculty of Social Sciences. We therefore invite researchers across the faculty to present ongoing research projects, project applications or just a loose idea that relates to the subject of social data science.
The rules are simple: short research presentations of ten minutes are followed by twenty minutes of debate. No papers will be circulated beforehand, and the presentations cannot be longer than five slides.
First discussion
Transforming Prediction Policy: How Novel Machine Learning Methods Can Improve Higher Education Admission
By: Magnus Lindgaard Nielsen, Jonas Skjold Raaschou-Pedersen, Emil Chrisander, Julien Grenet, Anna Rogers, David Dreyer Lassen, and Andreas Bjerre-Nielsen.
Presenter: Magnus Lindgaard Nielsen
Abstract: We investigate how recent advances in machine learning can improve algorithmic policy-making. We base our study in the context of admission to higher education in Denmark, where we exploit a unique large-scale dataset on the historic academic success of students and pre-admission information about them. We estimate a model based on the high-dimensional and sequential information encoded in the complex data of grade transcripts from elementary and high school by leveraging the transformer architecture. Our model improves the performance over standard machine learning models based on aggregate information from transcripts, irrespective of whether student background information is included. To assess our model’s potential for algorithmic policy-making, we embed it in a counterfactual admission prediction policy setup. We find that our model selects students with higher graduation rate compared to selection using actual admission criteria, either based on high school GPA or faculty evaluation. Our model satisfies the fairness concept sufficiency across a wide variety of sensitive attributes. We find that our models exhibit similar fairness properties compared to the current admission policies measured. Our results demonstrate the potential for using novel machine learning techniques to improve prediction policy-making using a new method of data representation for complex tabular data.
Second discussion
Modeling latent political views using LLM-embeddings
By: Tobias Priesholm Gårdhus, Jeppe Søndergaard Johansen, and August Lohse.
Presenter: Jeppe Søndergaard Johansen
Abstract: This paper considers how to use large language models when exploring latent structures of unstructured data such as text, when some information is available about the ordering of the authors’ views. To illustrate the capabilities of this method, we use it to ideologically scale Facebook posts from politicians, exploiting that we know their party affiliation to map LLM-embeddings of the posts into a 1-dimensional ideological representation. This 1-dimensional representation of the posts has a specific ordering and is restricted to follow a standard normal distribution, allowing for easy interpretation. Concretely, we construct a neural network that maps Facebook posts embeddings to a 1-dimensional layer from which we use ordered probit to predict party affiliation. We consider two distinct ways of restricting the latent space to a standard normal distribution. First, we consider KL-divergence akin to variational auto-encoders, second, we consider using insights from Stein's method to construct an adversarial estimation technique. More broadly we present a way to combine unstructured data (with ordered labels) into a structured representation, that can be used for further analysis. In our example, we consider ideological scaling, but could easily be extended to other use cases.