SODAS Data Discussion 10 November 2023
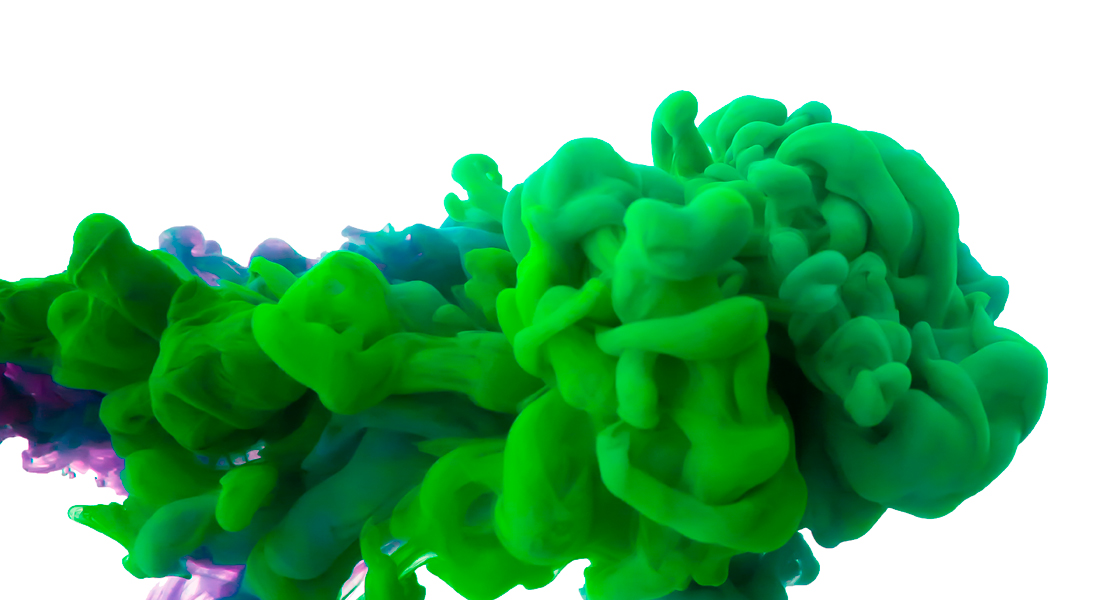
Copenhagen Center for Social Data Science (SODAS) aspirers to be a resource for all students and researchers at the Faculty of Social Sciences. We therefore invite researchers across the faculty to present ongoing research projects, project applications or just a loose idea that relates to the subject of social data science.
The rules are simple: short research presentations of ten minutes are followed by twenty minutes of debate. No papers will be circulated beforehand, and the presentations cannot be longer than five slides.
SODAS DATA DISCUSSION: SDS STUDENTS EDITION
Join us for November’s Data Discussion, which features two students from our Social Data Science program presenting ongoing work.
First discussion
Measuring the Rhetorical Mainstreaming of Radical Right Parties through Supervised Machine Learning
Presenter: Dario Ramon Landwehr
Abstract: Once insurgent outsiders, radical right parties in Europe are now in many cases entrenched features of national party systems. Many are now faced with the option of collaborating with mainstream parties in government, either as coalition partners or support parties. Can they do so while maintaining their radical edge? We provide new evidence on how radical right party rhetoric changes during and after government collaboration. Using machine learning methods applied to around 1.5 million parliamentary speeches across five countries, we develop a measure of the rhetorical distinctiveness of radical right parties at the speech level. We then estimate differential trends in distinctiveness for government collaborators using difference-in-differences models. We find that when collaborating with government parties, radical right parties consistently use less distinctive rhetoric. Distinctiveness rebounds after radical right parties stop collaborating. Our findings shed new light on the mainstreaming of the radical right and the dynamics driving party system change.
Second discussion
Behavioral Segmentation of Danish Video-On-Demand Streaming
By: Anders Saabye Møller
Abstract: What can be learned from segmenting the Video-On-Demand (VOD) streaming market in Denmark, and how may rich behavioral data be segmented? This industrial thesis, partnered with the Danish Broadcasting Corporation (DR), aims to pinpoint behavioral segments and understand how they engage with public service offerings. My dataset of 3,800 panelists tracks viewing across all devices, from major streaming platforms to social media, with more granular data for the Danish VOD streaming platforms. Though UMAP-HDBSCAN cluster analysis has been applied, it falls short in capturing data's longitudinal nuances—a gap potentially bridged by unsupervised learning methods like LSTM networks. I will present initial findings and welcome a discussion on the affordances of the data.