SODAS Data Discussion 1 December 2023
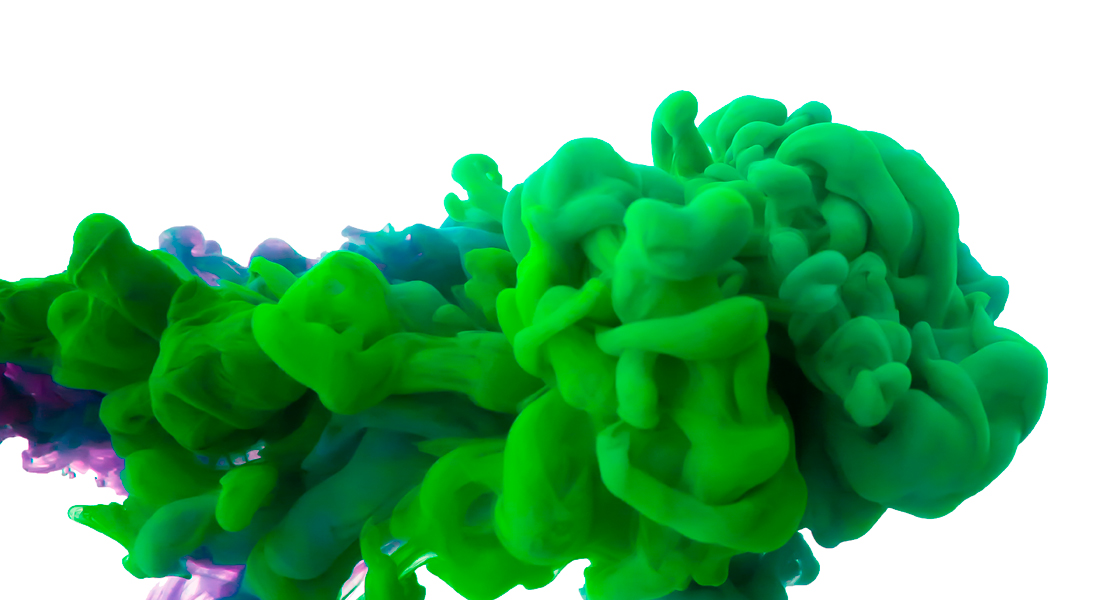
Copenhagen Center for Social Data Science (SODAS) aspirers to be a resource for all students and researchers at the Faculty of Social Sciences. We therefor invite researchers across the faculty to present ongoing research projects, project applications or just a loose idea that relates to the subject of social data science.
Two researchers will present their work. The rules are simple: short research presentations of ten minutes are followed by twenty minutes of debate. No papers will be circulated beforehand, and the presentations cannot be longer than five slides.
Discussion 1
Presenter: Fabian Beer.
Title: Explainability in the Field: How the »Black Box Problem« of AI is practically dealt with by a Predictive Policing Unit in Germany.
Abstract: The last few years have seen the rise of a research field by the name of Explainable AI (XAI). At the core of XAI lies the attempt to solve the so-called »Black Box problem«, a term that refers to the difficulty of providing an explanation for how exactly a machine learning (ML) model has arrived at an output. This problem, grounded in the opacity of the decision-making logic of some ML models, is said to be particularly acute in domains of “high-risk decision-making” such as military, finance, medicine or jurisdiction. In my presentation I will explore how the »Black Box problem« appears and is dealt with by the users themselves in one of these practical contexts: Predictive Policing. I will focus on a specific instance where a predictive policing unit in Germany, responsible for managing a random forest model that predicts burglaries in specific areas of the city, answered a request for an explanation of the model’s predictions by a local police department.
Discussion 2
Presenter: Elisabetta Salvai.
Title: Fair ranking by Biased random walk.
Abstract: Ranking algorithms play a significant role in ordering information in networks and identifying important and influential nodes. In this study, we investigate the fairness of the widely used PageRank algorithm in networks of nodes with binary attributes. We propose a new fairness definition rooted in demographic parity in the top-ranked positions, where the observer’s attention is predominantly concentrated. This definition is based on the idea that a fair ranking has the same proportion of attributes in the top-ranked positions as in the whole network. To improve the fairness of rankings, we then study a modification of the PageRank algorithm where we add a parameter that biases the random walk exploration at the core of the algorithm. This parameter changes the choice probability of the random walkers based on the degree of the neighbouring nodes. We study this biased PageRank algorithm, in both synthetic and real-word networks, for different values of the bias parameter. Our aim is to determine the effect of this targeted bias in enhancing ranking fairness.